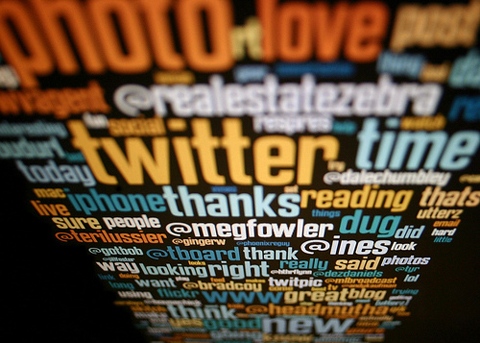
The internet and the web have changed the way we do business, learn, communicate, live and even think – a development apparent to many people. What is not so well known is that the internet has also started to change the scientific landscape in various – arguably profound – respects.
Rather than outline all these changes, I’m going to elaborate on one specific issue — one which really stands out, since it provides us with the chance to undertake completely novel scientific activity: “big data”. The term “big data” refers to very large sets – containing gigabytes of data and beyond – which can be accumulated from all over the internet, be it via online news, forum discussions, Youtube comments, product reviews, blog posts or social media traffic.
A very interesting aspect of this kind of data is that it is social data, produced by humans using the web as their medium of communication. It is the intrinsic accessibility and openness of the web that is essential in this respect: These qualities not only allow others to read, comment, reply or share what you write, post or upload – they also allow researchers to take a deeper look at what is actually ‘going on’. Often referred to as “Computational Social Science”, it is a newly emerging field with the possibility of substantially increasing and altering our understanding of how societies work.
The micro-blogging platform Twitter is one example of such a rich data source. Due to its open nature – everyone can read everything that is being publicly tweeted – it is an ideal and unique source for studying questions about human interaction, information diffusion, trend dynamics, political engagement and more.
In 2010, Alan Mislove and his fellow researchers at Northeastern and Harvard Universities were able to automatically detect and visualize geographical variations in mood-swings of the US Twitter community. The video below shows a time-lapse map of these ‘tweet moods’ on a color spectrum ranging from red to green, green being the happiest.
While it may seem fun and light-hearted, this kind of research is far from trivial: Detecting the moods expressed in millions of tweets requires serious computational algorithms able to do this task. These algorithms are called ‘sentiment classifiers’ and are the subject of recent research involving techniques from machine learning and artificial intelligence. One such classifier I could mention is SentiStrength, developed by a British research group as part of the European CyberEmotions project.
Diffusion and Interaction
Another interesting area of research is investigating how information spreads on Twitter. Why do some tweets become popular while others drift into obscurity? In a recent paper, researchers at ETH Zurich (including myself) identified a criterion for the success of a tweet being re-tweeted: Our analyses showed that tweets in which words with high emotional contrast — such as ‘hate’ and ‘love’ — occur next to each other are up to four times more likely to be re-tweeted. Emotions and politics often go together, and Twitter research has also ventured into this domain.
Twitter users’ political interactions were addressed by Michael D Conover et al in their 2011 paper. The researchers were able to pinpoint clusters of users with opposing political convictions and then identify interaction patterns between the groups. The remarkable finding was that re-tweeting mostly took place within a certain political group, whereas there was rather heavy @mention-traffic between the two partisan groups. One possible explanation is that individuals included small pieces of content — in this case a mention of a user from the other political side — in order to spread their own opinions into the opposing group and to provoke an interaction: Users get alerts when they are mentioned in a post and hence will most likely read it. This finding seems to confirm other research contesting the reciprocity hypothesis (also known as the ‘echo chamber theory’, it suggests that people tend to only interact online with people holding similar opinions rather than engage with those of different political, religious or other persuasions).
Future responsibilities
It’s not only social networks that provide plenty of research opportunities – so do interactions between people and technology in general. Most prominently, the search volume of specific search terms in Google, as provided by Google Trends, has been shown to be useful in monitoring and predicting the outbreak of influenza epidemics. This kind of ‘data-driven science’ will become even more important in the future as the boundaries between off-line and on-line blur, especially with the transition to what has been termed the ‘Internet of things’.
People should also be aware, however, that all this opportunity for exciting research (should) come with a sense of great responsibility on the part of researchers: It needs to be used only for non-malicious purposes, and with complete respect regarding users’ privacy. In general this is often not a problem for scientists – they are not interested in the particular individual but rather in more general behavior. However, it should be remembered that not everything which is scientifically feasible is also desirable.
René Pfitzner is a PhD student at the Chair of Systems Design at ETH
Zurich. A theoretical physics graduate, his research now focuses on complex interactions in socio-technical systems.